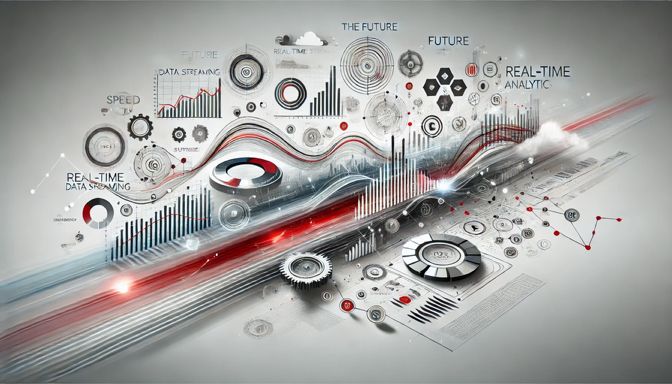
Below are the key trends and factors shaping the future of real-time data streaming:
Integration with AI and Machine Learning
Real-time analytics: Streaming data will power on-the-fly AI models for dynamic decision-making.
Predictive maintenance: Sensors and IoT devices will leverage real-time streams to predict and prevent equipment failures.
LLM applications: Chatbots and other AI services will use real-time data to provide more accurate and timely responses.
Evolution of Streaming Technologies
Platforms like Apache Kafka, Apache Pulsar, and AWS Kinesis will continue to mature.
Unified Batch and Streaming: Technologies like Apache Flink and Databricks Delta will enable seamless integration of real-time and batch processing.
Edge Computing: Real-time streaming at the edge will reduce latency and enable faster decision-making.
Expansion of IoT and 5G
IoT proliferation: Billions of connected devices will generate massive volumes of streaming data.
5G networks: Increased bandwidth and lower latency will enhance real-time streaming capabilities, enabling applications like autonomous vehicles and smart cities.
Focus on Scalability and Cost Efficiency
Cloud-based solutions will dominate, with providers like AWS, Azure, and GCP offering scalable, managed services for real-time streaming.
Open-source solutions will continue to be refined for lower-cost implementations.
Real-Time Data Governance and Security
Real-time governance frameworks will ensure compliance with data regulations like GDPR and CCPA.
Enhanced encryption and security protocols will protect sensitive data streams.
Emergence of Real-Time Digital Twins
Industries like manufacturing, energy, and urban planning will use real-time data streaming to create and update digital twins for simulation and optimization.
Serverless and Event-Driven Architectures
Event-driven frameworks will become the backbone of real-time systems, enabling applications to respond instantly to specific triggers.
Democratization of Real-Time Analytics
Advances in low-code/no-code platforms will allow more users, including non-technical stakeholders, to harness real-time data for business intelligence.
Sustainability and Energy Efficiency
Optimized algorithms and energy-efficient processing will make real-time streaming more sustainable, especially in large-scale IoT networks.
Challenges to Address
Latency Reduction: As applications like autonomous vehicles require ultra-low latency, improving processing speeds will be critical.
Data Quality: Ensuring real-time data is clean and reliable remains a challenge.
Interoperability: Seamless integration between various streaming platforms and enterprise systems is needed.